Rapid prediction of bacterial growth inhibition using Google's Coral AI platform
Every year waterborne diseases cause a substantial economic burden, costing more than $2 billion in treatments in the US alone. Roughly 90 million patients fall ill per year to conditions such as Escherichia coli (E. coli). E. coli, one of the most common public health concerns, is spread through drinking water, contaminated food consumption, and contact with infected animals or people. Recently, certain strains have become immune to Penicillin, a common antibiotic. Therefore, it must be detected early to avoid any infections by the super disease.
Several E. coli detection methods exist, such as culturing samples on solid agar plates or in liquid media. The use of liquid growth media provides high sensitivity; however, it requires at least 18 hours for the final read-out. Solid agar plates are more cost-effective and more flexible but often take 24 to 48 hours to grow. It is also possible to use molecular detection methods to reduce the assay time to a few hours; however, the results lack the sensitivity of the tests mentioned previously. There is a strong need for an automated method that can achieve rapid colony detection with high sensitivity to accelerate the identification of dangerous diseases in a laboratory setting.
To provide a powerful alternative that can rapidly detect and classify resistant vs. non-resistant E. coli, we propose a system that will collect live growth data of E. coli with which it will use to classify the bacteria into the two required categories. The system will be composed of a physical device to collect the visual data and a software component to detect and classify the bacteria. The device will be capable of accumulating a video feed of E. coli samples. The video will be of sufficient length and quality to obtain the most accurate predictions. Due to restrictions in the lab, the device is small and portable. The software component is composed of a runner program and an ML model. The results from our system will accelerate the detection of resistant E. coli by many hours, which can help avoid many infections and outbreaks.
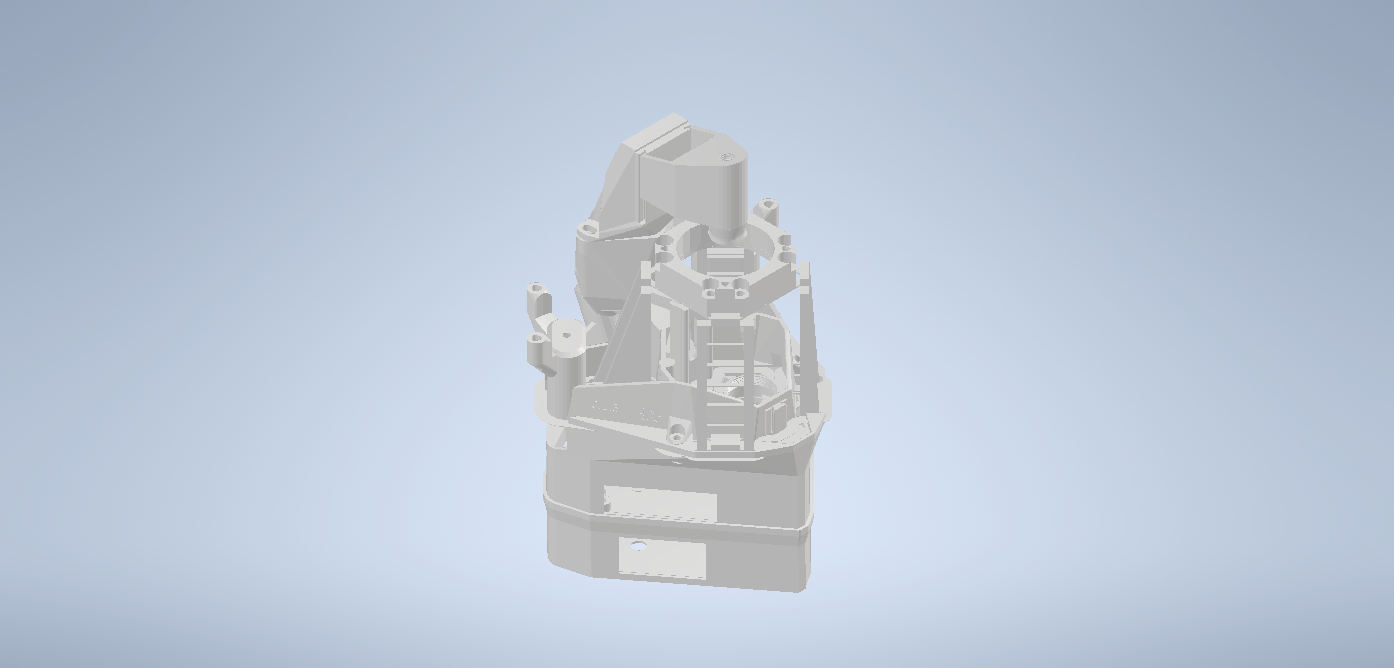